Data Warehousing
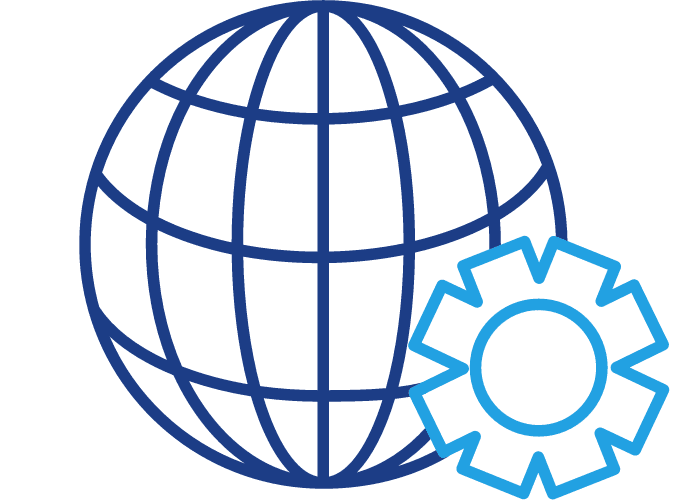
Building and using a data warehouse is known as data warehousing. Data from several heterogeneous sources are combined to create a data warehouse, which supports analytical reporting, structured and/or ad queries, and decision-making. Data cleansing, data integration, and data consolidation are all components of data warehousing.
डेटा वेयरहाउस का निर्माण और उपयोग करना डेटा वेयरहाउसिंग के रूप में जाना जाता है। डेटा वेयरहाउस बनाने के लिए कई विषम स्रोतों से डेटा को जोड़ा जाता है, जो विश्लेषणात्मक रिपोर्टिंग, संरचित और/या विज्ञापन प्रश्नों और निर्णय लेने का समर्थन करता है। डेटा क्लींजिंग, डेटा इंटीग्रेशन और डेटा समेकन डेटा वेयरहाउसिंग के सभी घटक हैं।
Using Data Warehouse Information
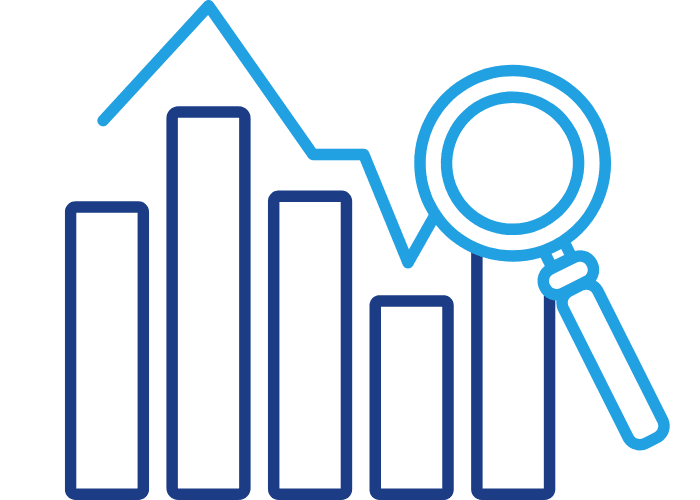
is possible to employ decision support technologies to make use of data that is stored in a data warehouse. Executives can utilize the warehouse more efficiently gently and swiftly thanks to this technology. They can collect information, examine it, and make judgments based on the data in the warehouse. Any of the following fields can make use of the data acquired in a warehouse:
डेटा वेयरहाउस में संग्रहीत डेटा का उपयोग करने के लिए निर्णय समर्थन तकनीकों को नियोजित करना संभव है। इस तकनीक की बदौलत कार्यकारी अधिकारी गोदाम का अधिक कुशलता से धीरे और तेजी से उपयोग कर सकते हैं। वे जानकारी एकत्र कर सकते हैं, इसकी जांच कर सकते हैं और गोदाम में डेटा के आधार पर निर्णय ले सकते हैं। निम्न में से कोई भी फ़ील्ड वेयरहाउस में प्राप्त डेटा का उपयोग कर सकता है:
Tuning Production Strategies
Repositioning the items and managing the product portfolios by comparing the sales quarterly or yearly might help to fine-tune the product strategy.
बिक्री की त्रैमासिक या वार्षिक तुलना करके वस्तुओं की स्थिति बदलने और उत्पाद पोर्टफोलियो का प्रबंधन करने से उत्पाद रणनीति को ठीक करने में मदद मिल सकती है।
Customer Analysis
Customer analysis involves looking at the customer’s purchasing habits, budget cycles, and other factors.
ग्राहक विश्लेषण में ग्राहक की खरीदारी की आदतों, बजट चक्रों और अन्य कारकों को देखना शामिल है।
Operations Analysis
In addition, data warehousing supports environmental improvements and customer relationship management. We can examine corporate activities thanks to the information.
इसके अलावा, डेटा वेयरहाउसिंग पर्यावरण सुधार और ग्राहक संबंध प्रबंधन का समर्थन करता है। हम जानकारी के लिए कॉर्पोरेट गतिविधियों की जांच कर सकते हैं।
Integrating Heterogeneous Databases

Query-driven Approach
The conventional method of integrating disparate datasets is as described above. On top of several heterogeneous databases, wrappers, and integrators were built using this methodology. These mediators are another name for these integrators.
अलग-अलग डेटासेट को एकीकृत करने की पारंपरिक विधि ऊपर बताई गई है। इस पद्धति का उपयोग करके कई विषम डेटाबेस, रैपर और इंटीग्रेटर्स के ऊपर बनाया गया था। ये मध्यस्थ इन इंटीग्रेटर्स का दूसरा नाम हैं।
Data Warehousing And Data Mining
Process of Query-Driven Approach
A metadata dictionary converts a query into the proper form for each of the concerned individual heterogeneous sites when it is sent to a client side.
एक मेटाडेटा डिक्शनरी एक ग्राहक पक्ष को भेजे जाने पर संबंधित व्यक्तिगत विषम साइटों में से प्रत्येक के लिए एक क्वेरी को उचित रूप में परिवर्तित करती है।
These requests are now mapped and delivered to the neighborhood query processor.
इन अनुरोधों को अब मैप किया जाता है और पड़ोस के क्वेरी प्रोसेसर को डिलीवर किया जाता है।
A global answer set incorporates the findings from several places.
एक वैश्विक उत्तर सेट में कई स्थानों के निष्कर्ष शामिल होते हैं।
Disadvantages
Complex integration and filtering procedures are required for query-driven approaches.
This strategy is quite ineffective.
In case of repeated requests, it is highly costly.
For queries that call for aggregation, this strategy is also quite costly.
क्वेरी-संचालित दृष्टिकोणों के लिए जटिल एकीकरण और फ़िल्टरिंग प्रक्रियाओं की आवश्यकता होती है।
यह रणनीति काफी अप्रभावी है।
बार-बार अनुरोध के मामले में, यह अत्यधिक महंगा है।
एकत्रीकरण की मांग करने वाले प्रश्नों के लिए, यह रणनीति काफी महंगी भी है।
Data mining
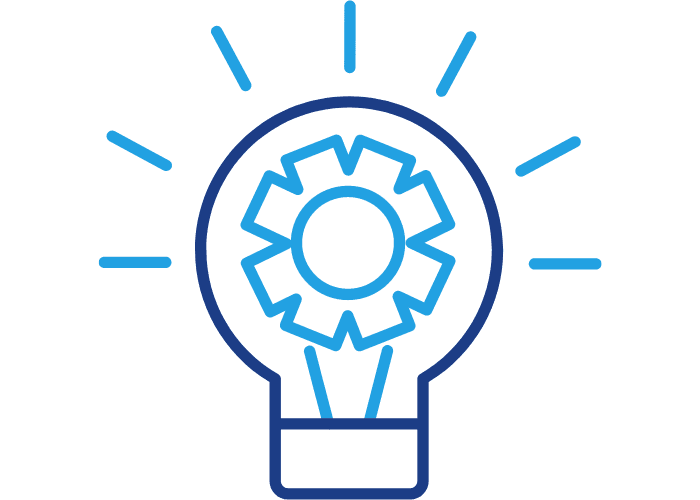
Using algorithms and statistical models, data mining is the process of extracting patterns, correlations, and insights from massive volumes of data. The purpose of data mining is to take useful information from data and organize it in a way that may be used later.
एल्गोरिदम और सांख्यिकीय मॉडल का उपयोग करते हुए, डेटा माइनिंग बड़े पैमाने पर डेटा से पैटर्न, सहसंबंध और अंतर्दृष्टि निकालने की प्रक्रिया है। डेटा माइनिंग का उद्देश्य डेटा से उपयोगी जानकारी लेना और उसे इस तरह से व्यवस्थित करना है जिसका उपयोग बाद में किया जा सके।
Process Of Data Mining
Data preparation includes preparing the data for analysis by cleaning, converting, and pre-processing it.
डेटा तैयारी में डेटा की सफाई, रूपांतरण और पूर्व-प्रसंस्करण द्वारा विश्लेषण के लिए डेटा तैयार करना शामिल है।
Data preparation includes preparing the data for analysis by cleaning, converting, and pre-processing it.
डेटा तैयारी में डेटा की सफाई, रूपांतरण और पूर्व-प्रसंस्करण द्वारा विश्लेषण के लिए डेटा तैयार करना शामिल है।
Data exploration is the process of visualizing and summarising data to understand its features and spot any possible issues.
डेटा एक्सप्लोरेशन डेटा की विशेषताओं को समझने और किसी भी संभावित मुद्दों को समझने के लिए डेटा को विज़ुअलाइज़ करने और सारांशित करने की प्रक्रिया है।
Data modeling is the process of analyzing data to find patterns and correlations using statistical and machine learning methods.
डेटा मॉडलिंग सांख्यिकीय और मशीन सीखने के तरीकों का उपयोग करके पैटर्न और सहसंबंध खोजने के लिए डेटा का विश्लेषण करने की प्रक्रिया है।
Evaluation of patterns: Choosing the most important patterns from the data and assessing their reliability and applicability.
पैटर्न का मूल्यांकन: डेटा से सबसे महत्वपूर्ण पैटर्न चुनना और उनकी विश्वसनीयता और प्रयोज्यता का आकलन करना।
Knowledge representation is the process of transforming patterns into understandable and practical forms, such as rules, decision trees, or graphs.
ज्ञान प्रतिनिधित्व पैटर्न को समझने योग्य और व्यावहारिक रूपों में बदलने की प्रक्रिया है, जैसे नियम, निर्णय वृक्ष या ग्राफ़।
Deployment: Putting the outcomes of data mining into a production system, such as a database, data warehouse, or decision support system.
परिनियोजन: डेटा माइनिंग के परिणामों को एक प्रोडक्शन सिस्टम, जैसे डेटाबेस, डेटा वेयरहाउस, या डिसीजन सपोर्ट सिस्टम में डालना।
Monitoring: Keeping tabs on the data and the outcomes of the data mining to make sure they continue to be correct and pertinent
निगरानी: यह सुनिश्चित करने के लिए डेटा और डेटा माइनिंग के परिणामों पर नज़र रखना कि वे सही और प्रासंगिक बने रहें
Data Mining Pre-Processing
Data Cleaning: Removing missing values, duplicate records, and inconsistent or irrelevant data.
डेटा क्लीनिंग: लापता मान, डुप्लिकेट रिकॉर्ड और असंगत या अप्रासंगिक डेटा को हटाना।
Data Transformation: Converting data into a standardized format, such as normalizing numerical values, converting categorical data into numerical data, etc.
डेटा परिवर्तन: डेटा को एक मानकीकृत प्रारूप में परिवर्तित करना, जैसे संख्यात्मक मानों को सामान्य करना, श्रेणीबद्ध डेटा को संख्यात्मक डेटा में परिवर्तित करना आदि।
Data Integration: Combining multiple data sources into a single unified data set.
डेटा एकीकरण: एक ही एकीकृत डेटा सेट में कई डेटा स्रोतों का संयोजन।
Data Reduction: Reducing the data volume by aggregating data or reducing the dimensionality of data through feature selection or feature extraction.
डेटा विकेंद्रीकरण: संख्यात्मक डेटा को अंतराल या डिब्बे में विभाजित करके श्रेणीबद्ध डेटा में परिवर्तित करना।
Data Discretization: Converting numerical data into categorical data by dividing it into intervals or bins.
डेटा में कमी: डेटा एकत्र करके डेटा की मात्रा को कम करना या सुविधा चयन या सुविधा निष्कर्षण के माध्यम से डेटा के आयाम को कम करना।